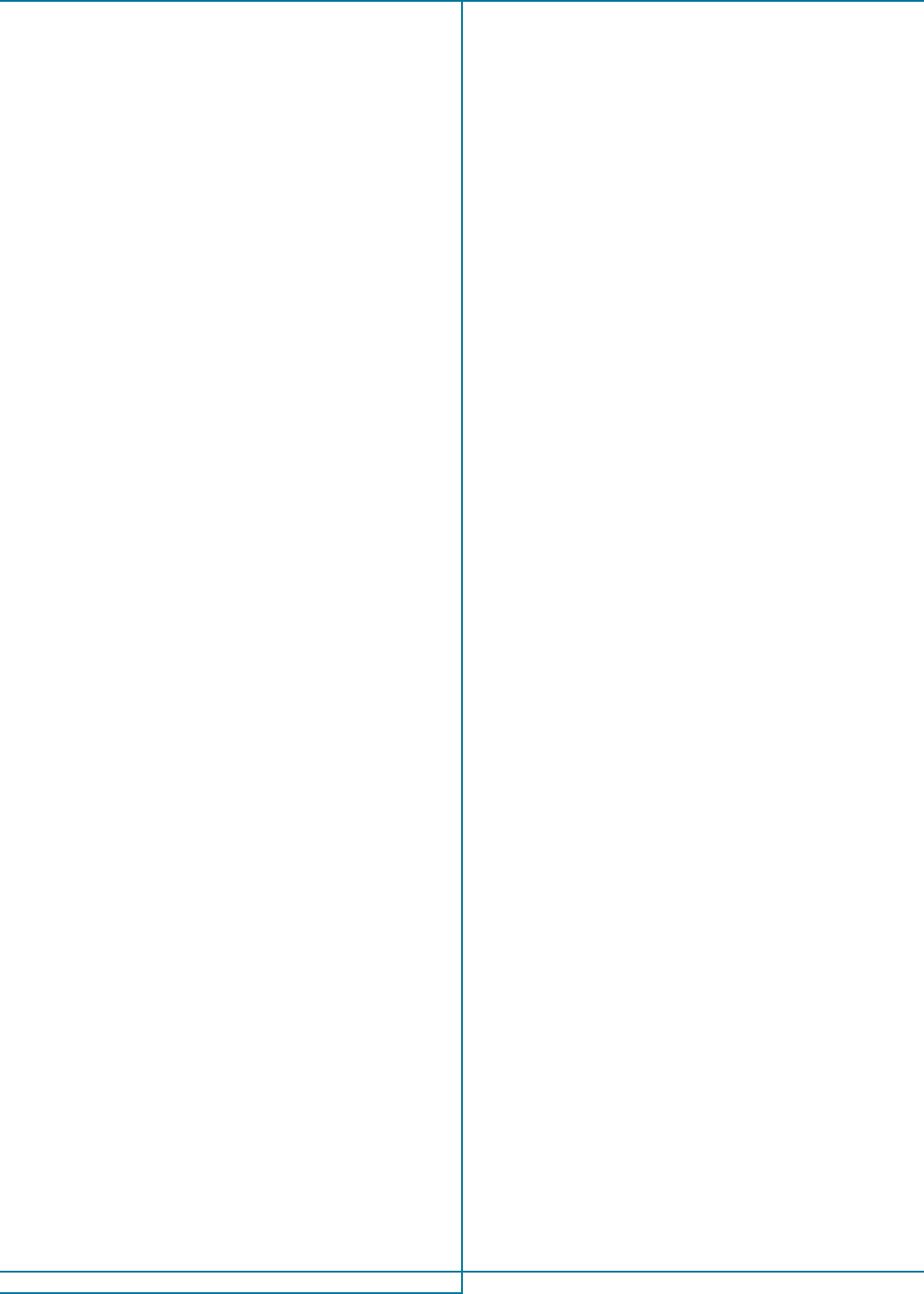
36 The Role of Bank Credit Enhancements in Securitization
the level of enhancements on a deal and the performance of
securitized assets. Note that in this scenario, enhancements
serve as a buffer against observable risk (as embodied in the
estimated loss function).
We are interested in the idea that enhancements might also
be used to solve part of the asymmetric information problems
that may plague the securitization process. If banks are better
informed than outside investors about the quality of the assets
they are securitizing, as they almost certainly are, banks that are
securitizing higher-quality assets may use enhancements as a
signal of their quality. In other words, by their willingness to
keep “skin in the game” to retain some risk, banks can signal
their faith in the quality of their assets. Such signaling implies
a positive relationship between the level of enhancements and
the performance of securitized assets, just the opposite of the
buffer explanation. Obviously, enhancements could, and
probably do, serve both as a buffer against observable risk
and a signal against unobservable (to outsiders) quality.
However, since the buffer role is almost self-evidently true,
we are interested in whether we can detect any evidence for
the role of securitization enhancements as a signal.
Others have also considered the hypothesis that
enhancements might play a signaling role. Downing, Jaffee,
and Wallace (2009) observe that asymmetric information
about prepayment risk in the government-sponsored-
enterprise (GSE) mortgage-backed-security market should
motivate the use of signaling devices.
3
Albertazzi et al. (2011)
note the potential centrality of asymmetric information to
the securitization process and conjecture that a securitizing
sponsor can keep a junior (equity) tranche “as a signaling”
device of its (unobservable) quality or as an expression of a
commitment to continue monitoring. James (2010) comments
that if asset-backed securities include a moral hazard (or
“lemons”) discount due to asymmetric information, issuers
have an incentive to retain some risk “as a way of
demonstrating higher underwriting standards.”
4
A variant of the question we are asking about credit
enhancements showed up in earlier literature on the role
of collateral in traditional (on-the-books) bank lending.
A theoretical literature in the 1980s predicted that in the
context of asymmetric information, safer borrowers were more
likely to pledge collateral to distinguish themselves from riskier
ones (Besanko and Thakor 1987; Chan and Kanatas 1985).
However, an empirical study by Berger and Udell (1990) found
strong evidence against the signaling hypothesis: that is,
3
Because the mortgage-backed securities that the authors study are
guaranteed, prepayment risk is the only risk investors need to worry about.
4
In a paper that is somewhat related to ours, Erel, Nadauld, and Stulz (2011,
p. 37) investigate why banks hold highly rated tranches of securitizations,
and conclude that their doing so may partly serve as “a credible signal of deal
quality to potential investors.”
collateral was associated with riskier borrowers and loans.
In other words, when it comes to loans on the books, collateral
seems to serve more as a buffer against observable risk than
as a signal of unobservable quality.
We found only one other paper that looks at the relationship
between enhancements and the performance of securitized
assets. Using loan-level data, Ashcraft, Vickery, and
Goldsmith-Pinkham (2010) find that delinquency on
underlying subprime and Alt-A mortgage pools is positively
associated with the amount of AAA subordination.
5
Those
results are consistent with the hypothesis that subordination is
used as a buffer against observable credit risk. Interestingly,
however, the authors find that BBB subordination is negatively
associated with mortgage performance on Alt-A deals, which
they consider more opaque (hard to rate). The latter result
seems consistent with the signaling hypothesis: the issuer of an
opaque security submits to a high degree of subordination to
signal its confidence in the quality of the assets it is selling.
We investigate our question from two angles. First, we look
directly at the relationship between the performance of
securitized assets and total enhancements in a panel analysis
where we regress the fraction of securitized assets that are
severely delinquent (delinquent for ninety or more days or
charged off) on total enhancements per unit of securitized
assets. We estimate the regression for seven categories of credit:
residential real estate loans, home equity loans, credit card
loans, auto loans, other consumer loans, all other loans, and
total securitizations. We are not able to detect any evidence
for the signaling hypothesis; when we find a significant
relationship between delinquency on securitized assets and
enhancements, the relationship is positive, consistent with
the buffer hypothesis.
In the second part of our article, we test the hypotheses
from the perspective of market participants. Specifically,
we investigate how stock investors and the option market
reacted when BHCs detailed for the first time their
securitization activity in their 2001:Q2 regulatory reports,
which include enhancements and aggregate loan performance
(delinquencies) of the assets that BHCs securitized. We
calculate the cumulative abnormal stock return around that
date for each BHC that had positive securitization activity.
We find first that abnormal returns are highly positively
correlated with the extent of securitization activity at a
BHC. That comes as no surprise, since securitization was
presumably viewed at the time as positive net-present-value
(NPV) activity. More interestingly, we find that the
relationship between total credit enhancements and
5
The amount of subordination at a given rating is the fraction of bonds that
absorb losses before the bond in question. If 90 percent of the bonds in a deal
are senior AAA bonds and 10 percent are junior, subordination of the AAA
bonds is 10 percent.